1/2013 - 15 |
Segmentation of Bone Structure in X-ray Images using Convolutional Neural NetworkCERNAZANU-GLAVAN, C.![]() ![]() ![]() ![]() ![]() ![]() |
Extra paper information in ![]() ![]() ![]() |
Click to see author's profile in ![]() ![]() ![]() |
Download PDF ![]() |
Author keywords
image segmentation, neural network, convolution, biomedical image processing
References keywords
neural(11), recognition(9), segmentation(8), networks(8), images(6), schmidhuber(5), image(5), medical(4), cvpr(4), convolutional(4)
Blue keywords are present in both the references section and the paper title.
About this article
Date of Publication: 2013-02-28
Volume 13, Issue 1, Year 2013, On page(s): 87 - 94
ISSN: 1582-7445, e-ISSN: 1844-7600
Digital Object Identifier: 10.4316/AECE.2013.01015
Web of Science Accession Number: 000315768300015
SCOPUS ID: 84875328950
Abstract
The segmentation process represents a first step necessary for any automatic method of extracting information from an image. In the case of X-ray images, through segmentation we can differentiate the bone tissue from the rest of the image. There are nowadays several segmentation techniques, but in general, they all require the human intervention in the segmentation process. Consequently, this article proposes a new segmentation method for the X-ray images using a Convolutional Neural Network (CNN). In present, the convolutional networks are the best techniques for image segmentation. This fact is demonstrated by their wide usage in all the fields, including the medical one. As the X-ray images have large dimensions, for reducing the training time, the method proposed by the present article selects only certain areas (maximum interest areas) from the entire image. The neural network is used as pixel classifier thus causing the label of each pixel (bone or none-bone) from a raw pixel values in a square area. We will also present the method through which the network final configuration was chosen and we will make a comparative analysis with other 3 CNN configurations. The network chosen by us obtained the best results for all the evaluation metrics used, i.e. warping error, rand error and pixel error. |
References | | | Cited By |
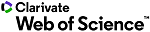
Web of Science® Times Cited: 51 [View]
View record in Web of Science® [View]
View Related Records® [View]
Updated today
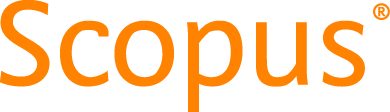
SCOPUS® Times Cited: 79
View record in SCOPUS® [Free preview]
View citations in SCOPUS® [Free preview]
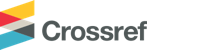
[1] A hybrid deep learning approach using XceptionNet and vision transformer for accurate chest disease detection from X-ray images, Bhuvanya, R., Vanitha, V., Iqbal, M.Mohamed, Dumka, Ankur, Singh, Rajesh, Gehlot, Anita, Thakur, Amit Kumar, Biomedical Signal Processing and Control, ISSN 1746-8094, Issue , 2025.
Digital Object Identifier: 10.1016/j.bspc.2025.108118 [CrossRef]
[2] Diagnosis of Osteoporosis using modified U-net architecture with attention unit in DEXA and X-ray images, Nazia Fathima, S.M., Tamilselvi, R., Parisa Beham, M., Sabarinathan, D., Journal of X-Ray Science and Technology: Clinical Applications of Diagnosis and Therapeutics, ISSN 0895-3996, Issue 5, Volume 28, 2020.
Digital Object Identifier: 10.3233/XST-200692 [CrossRef]
[3] Skin lesion segmentation in dermoscopy images via deep full resolution convolutional networks, Al-masni, Mohammed A., Al-antari, Mugahed A., Choi, Mun-Taek, Han, Seung-Moo, Kim, Tae-Seong, Computer Methods and Programs in Biomedicine, ISSN 0169-2607, Issue , 2018.
Digital Object Identifier: 10.1016/j.cmpb.2018.05.027 [CrossRef]
[4] Multiscale sequential convolutional neural networks for simultaneous detection of fovea and optic disc, Al-Bander, Baidaa, Al-Nuaimy, Waleed, Williams, Bryan M., Zheng, Yalin, Biomedical Signal Processing and Control, ISSN 1746-8094, Issue , 2018.
Digital Object Identifier: 10.1016/j.bspc.2017.09.008 [CrossRef]
[5] Artificial intelligence and the radiologist: the future in the Armed Forces Medical Services, Sen, Debraj, Chakrabarti, R, Chatterjee, S, Grewal, D S, Manrai, K, BMJ Military Health, ISSN 2633-3767, Issue 4, Volume 166, 2020.
Digital Object Identifier: 10.1136/jramc-2018-001055 [CrossRef]
[6] FractureNet: A Fusion Approach for Automated Hand Fracture Detection and Classification, M, Priyanka, Reddy, Chethan L, Aymon, Umme, Jipeng, Tian, Procedia Computer Science, ISSN 1877-0509, Issue , 2025.
Digital Object Identifier: 10.1016/j.procs.2025.04.348 [CrossRef]
[7] The importance of resource awareness in artificial intelligence for healthcare, Jia, Zhenge, Chen, Jianxu, Xu, Xiaowei, Kheir, John, Hu, Jingtong, Xiao, Han, Peng, Sui, Hu, Xiaobo Sharon, Chen, Danny, Shi, Yiyu, Nature Machine Intelligence, ISSN 2522-5839, Issue 7, Volume 5, 2023.
Digital Object Identifier: 10.1038/s42256-023-00670-0 [CrossRef]
[8] Simultaneous detection and classification of breast masses in digital mammograms via a deep learning YOLO-based CAD system, Al-masni, Mohammed A., Al-antari, Mugahed A., Park, Jeong-Min, Gi, Geon, Kim, Tae-Yeon, Rivera, Patricio, Valarezo, Edwin, Choi, Mun-Taek, Han, Seung-Moo, Kim, Tae-Seong, Computer Methods and Programs in Biomedicine, ISSN 0169-2607, Issue , 2018.
Digital Object Identifier: 10.1016/j.cmpb.2018.01.017 [CrossRef]
[9] Statistical and Physical Micro-Feature-Based Segmentation of Cortical Bone Images Using Artificial Intelligence, Hage, Ilige S., Hamade, Ramsey, Materials Science Forum, ISSN 1662-9752, Issue , 2014.
Digital Object Identifier: 10.4028/www.scientific.net/MSF.783-786.222 [CrossRef]
[10] Skin lesion segmentation from dermoscopic images by using Mask R-CNN, Retina-Deeplab, and graph-based methods, Bagheri, Fatemeh, Tarokh, Mohammad Jafar, Ziaratban, Majid, Biomedical Signal Processing and Control, ISSN 1746-8094, Issue , 2021.
Digital Object Identifier: 10.1016/j.bspc.2021.102533 [CrossRef]
[11] Convolutional Neural Network-Based Humerus Segmentation and Application to Bone Mineral Density Estimation from Chest X-ray Images of Critical Infants, Liu, Yung-Chun, Lin, Yung-Chieh, Tsai, Pei-Yin, Iwata, Osuke, Chuang, Chuew-Chuen, Huang, Yu-Han, Tsai, Yi-Shan, Sun, Yung-Nien, Diagnostics, ISSN 2075-4418, Issue 12, Volume 10, 2020.
Digital Object Identifier: 10.3390/diagnostics10121028 [CrossRef]
[12] Knee Osteoarthritis Detection Using an Improved CenterNet With Pixel-Wise Voting Scheme, Aladhadh, Suliman, Mahum, Rabbia, IEEE Access, ISSN 2169-3536, Issue , 2023.
Digital Object Identifier: 10.1109/ACCESS.2023.3247502 [CrossRef]
[13] A perspective on the diagnosis of cracked tooth: imaging modalities evolve to AI-based analysis, Guo, Juncheng, Wu, Yuyan, Chen, Lizhi, Long, Shangbin, Chen, Daqi, Ouyang, Haibing, Zhang, Chunliang, Tang, Yadong, Wang, Wenlong, BioMedical Engineering OnLine, ISSN 1475-925X, Issue 1, Volume 21, 2022.
Digital Object Identifier: 10.1186/s12938-022-01008-4 [CrossRef]
[14] Bone shadow segmentation from ultrasound data for orthopedic surgery using GAN, Alsinan, Ahmed Z., Patel, Vishal M., Hacihaliloglu, Ilker, International Journal of Computer Assisted Radiology and Surgery, ISSN 1861-6410, Issue 9, Volume 15, 2020.
Digital Object Identifier: 10.1007/s11548-020-02221-z [CrossRef]
[15] Development of an Automated Mass-Customization Pipeline for Knee Replacement Surgery Using Biplanar X-Rays, Burge, Thomas A., Jeffers, Jonathan R. T., Myant, Connor W., Journal of Mechanical Design, ISSN 1050-0472, Issue 2, Volume 144, 2022.
Digital Object Identifier: 10.1115/1.4052192 [CrossRef]
[16] Deep Learning for Automatic Segmentation of Oral and Oropharyngeal Cancer Using Narrow Band Imaging: Preliminary Experience in a Clinical Perspective, Paderno, Alberto, Piazza, Cesare, Del Bon, Francesca, Lancini, Davide, Tanagli, Stefano, Deganello, Alberto, Peretti, Giorgio, De Momi, Elena, Patrini, Ilaria, Ruperti, Michela, Mattos, Leonardo S., Moccia, Sara, Frontiers in Oncology, ISSN 2234-943X, Issue , 2021.
Digital Object Identifier: 10.3389/fonc.2021.626602 [CrossRef]
[17] A computational tool for automatic selection of total knee replacement implant size using X-ray images, Burge, Thomas A., Jones, Gareth G., Jordan, Christopher M., Jeffers, Jonathan R.T., Myant, Connor W., Frontiers in Bioengineering and Biotechnology, ISSN 2296-4185, Issue , 2022.
Digital Object Identifier: 10.3389/fbioe.2022.971096 [CrossRef]
[18] DenseNet for Anatomical Brain Segmentation, Gottapu, Ram Deepak, Dagli, Cihan H, Procedia Computer Science, ISSN 1877-0509, Issue , 2018.
Digital Object Identifier: 10.1016/j.procs.2018.10.327 [CrossRef]
[19] Dual deterministic model based on deep neural network for the classification of pneumonia, Mustafa Khan, Muhammad, UI Islam, Muhammad Saif, Siddiqui, Ali Akbar, Qadri, Muhammad Tahir, Intelligent Decision Technologies, ISSN 1872-4981, Issue 3, Volume 17, 2023.
Digital Object Identifier: 10.3233/IDT-220192 [CrossRef]
[20] Mumford–Shah Loss Functional for Image Segmentation With Deep Learning, Kim, Boah, Ye, Jong Chul, IEEE Transactions on Image Processing, ISSN 1057-7149, Issue , 2020.
Digital Object Identifier: 10.1109/TIP.2019.2941265 [CrossRef]
[21] Digital Rock Segmentation for Petrophysical Analysis With Reduced User Bias Using Convolutional Neural Networks, Niu, Yufu, Mostaghimi, Peyman, Shabaninejad, Mehdi, Swietojanski, Pawel, Armstrong, Ryan T., Water Resources Research, ISSN 0043-1397, Issue 2, Volume 56, 2020.
Digital Object Identifier: 10.1029/2019WR026597 [CrossRef]
[22] Renal tumors segmentation in abdomen CT Images using 3D-CNN and ConvLSTM, Kang, Li, Zhou, Ziqi, Huang, Jianjun, Han, Wenzhong, Biomedical Signal Processing and Control, ISSN 1746-8094, Issue , 2022.
Digital Object Identifier: 10.1016/j.bspc.2021.103334 [CrossRef]
[23] Automatic delineation of ribs and clavicles in chest radiographs using fully convolutional DenseNets, Liu, Yunbi, Zhang, Xiao, Cai, Guangwei, Chen, Yingyin, Yun, Zhaoqiang, Feng, Qianjin, Yang, Wei, Computer Methods and Programs in Biomedicine, ISSN 0169-2607, Issue , 2019.
Digital Object Identifier: 10.1016/j.cmpb.2019.105014 [CrossRef]
[24] Segmentation of carotid arterial walls using neural networks, Samber, Daniel D, Ramachandran, Sarayu, Sahota, Anoop, Naidu, Sonum, Pruzan, Alison, Fayad, Zahi A, Mani, Venkatesh, World Journal of Radiology, ISSN 1949-8470, Issue 1, Volume 12, 2020.
Digital Object Identifier: 10.4329/wjr.v12.i1.1 [CrossRef]
[25] A two-step convolutional neural network based computer-aided detection scheme for automatically segmenting adipose tissue volume depicting on CT images, Wang, Yunzhi, Qiu, Yuchen, Thai, Theresa, Moore, Kathleen, Liu, Hong, Zheng, Bin, Computer Methods and Programs in Biomedicine, ISSN 0169-2607, Issue , 2017.
Digital Object Identifier: 10.1016/j.cmpb.2017.03.017 [CrossRef]
[26] A comprehensive review of clustering techniques in artificial intelligence for knowledge discovery: Taxonomy, challenges, applications and future prospects, Singh, Jaswinder, Singh, Damanpreet, Advanced Engineering Informatics, ISSN 1474-0346, Issue , 2024.
Digital Object Identifier: 10.1016/j.aei.2024.102799 [CrossRef]
[27] An approach for chest tube detection in chest radiographs, Mercan, Cem Ahmet, Celebi, Mustafa Serdar, IET Image Processing, ISSN 1751-9659, Issue 2, Volume 8, 2014.
Digital Object Identifier: 10.1049/iet-ipr.2013.0239 [CrossRef]
[28] Detection of concealed cars in complex cargo X-ray imagery using Deep Learning, Jaccard, Nicolas, Rogers, Thomas W., Morton, Edward J., Griffin, Lewis D., Journal of X-Ray Science and Technology: Clinical Applications of Diagnosis and Therapeutics, ISSN 0895-3996, Issue 3, Volume 25, 2017.
Digital Object Identifier: 10.3233/XST-16199 [CrossRef]
[29] Automated correlative segmentation of large Transmission X-ray Microscopy (TXM) tomograms using deep learning, Shashank Kaira, C., Yang, Xiaogang, De Andrade, Vincent, De Carlo, Francesco, Scullin, William, Gursoy, Doga, Chawla, Nikhilesh, Materials Characterization, ISSN 1044-5803, Issue , 2018.
Digital Object Identifier: 10.1016/j.matchar.2018.05.053 [CrossRef]
[30] Automatic 3D liver location and segmentation via convolutional neural network and graph cut, Lu, Fang, Wu, Fa, Hu, Peijun, Peng, Zhiyi, Kong, Dexing, International Journal of Computer Assisted Radiology and Surgery, ISSN 1861-6410, Issue 2, Volume 12, 2017.
Digital Object Identifier: 10.1007/s11548-016-1467-3 [CrossRef]
[31] Deep Learning in Medical Imaging: General Overview, Lee, June-Goo, Jun, Sanghoon, Cho, Young-Won, Lee, Hyunna, Kim, Guk Bae, Seo, Joon Beom, Kim, Namkug, Korean Journal of Radiology, ISSN 1229-6929, Issue 4, Volume 18, 2017.
Digital Object Identifier: 10.3348/kjr.2017.18.4.570 [CrossRef]
[32] Two-stage hemoglobin prediction based on prior causality, Chen, Yuwen, Zhong, Kunhua, Zhu, Yiziting, Sun, Qilong, Frontiers in Public Health, ISSN 2296-2565, Issue , 2022.
Digital Object Identifier: 10.3389/fpubh.2022.1079389 [CrossRef]
[33] Lung Nodule CT Image Segmentation Model Based on Multiscale Dense Residual Neural Network, Zhang, Xinying, Kong, Shanshan, Han, Yang, Xie, Baoshan, Liu, Chunfeng, Mathematics, ISSN 2227-7390, Issue 6, Volume 11, 2023.
Digital Object Identifier: 10.3390/math11061363 [CrossRef]
[34] Automatic segmentation of bone surfaces from ultrasound using a filter-layer-guided CNN, Alsinan, Ahmed Z., Patel, Vishal M., Hacihaliloglu, Ilker, International Journal of Computer Assisted Radiology and Surgery, ISSN 1861-6410, Issue 5, Volume 14, 2019.
Digital Object Identifier: 10.1007/s11548-019-01934-0 [CrossRef]
[35] A high-throughput framework for predicting three-dimensional structural–mechanical relationships of human cranial bones using a deep learning-based method, Guo, Weihao, Rezasefat, Mohammad, Rabey, Karyne N., Ouellet, Simon, Westover, Lindsey, Hogan, James David, Journal of the Mechanical Behavior of Biomedical Materials, ISSN 1751-6161, Issue , 2025.
Digital Object Identifier: 10.1016/j.jmbbm.2025.107007 [CrossRef]
[36] Correlation of Bone Textural Parameters with Age in the Context of Orthopedic X-ray Studies, Kamiński, Paweł, Obuchowicz, Rafał, Stępień, Aleksandra, Lasek, Julia, Pociask, Elżbieta, Piórkowski, Adam, Applied Sciences, ISSN 2076-3417, Issue 11, Volume 13, 2023.
Digital Object Identifier: 10.3390/app13116618 [CrossRef]
[37] Iterative approach for 3D reconstruction of the femur from un-calibrated 2D radiographic images, Youn, Kibeom, Park, Moon Seok, Lee, Jehee, Medical Engineering & Physics, ISSN 1350-4533, Issue , 2017.
Digital Object Identifier: 10.1016/j.medengphy.2017.08.016 [CrossRef]
[38] Discriminative feature extraction from X-ray images using deep convolutional neural networks, Srinivas, M., Roy, Debaditya, Mohan, C. Krishna, 2016 IEEE International Conference on Acoustics, Speech and Signal Processing (ICASSP), ISBN 978-1-4799-9988-0, 2016.
Digital Object Identifier: 10.1109/ICASSP.2016.7471809 [CrossRef]
[39] Retracted on March 1, 2022 : Vertebrae Segmentation from X-ray Images Using Convolutional Neural Network, Kuok, Chan-Pang, Fu, Min-Jun, Lin, Chii-Jen, Horng, Ming-Huwi, Sun, Yung-Nien, Proceedings of the 2018 International Conference on Information Hiding and Image Processing, ISBN 9781450365468, 2018.
Digital Object Identifier: 10.1145/3292425.3293463 [CrossRef]
[40] CCS Coding of Discharge Diagnoses via Deep Neural Networks, Helwe, Chadi, Elbassuoni, Shady, Geha, Mirabelle, Hitti, Eveline, Makhlouf Obermeyer, Carla, Proceedings of the 2017 International Conference on Digital Health, ISBN 9781450352499, 2017.
Digital Object Identifier: 10.1145/3079452.3079498 [CrossRef]
[41] Multi-Scale Network Based on Dilated Convolution for Bladder Tumor Segmentation of Two-Dimensional MRI Images, Xu, Jinyang, Kang, Li, Han, Wenzhong, Jiang, Jinwan, Zhou, Ziqi, Huang, Jianjun, Zhang, Tijiang, 2020 15th IEEE International Conference on Signal Processing (ICSP), ISBN 978-1-7281-4479-5, 2020.
Digital Object Identifier: 10.1109/ICSP48669.2020.9320972 [CrossRef]
[42] Detection of Knee Osteoarthritis Using the Xception Model and Voting Classifier, Rifat, Saima, Mohammed, Sheena, 2024 2nd International Conference on Artificial Intelligence Trends and Pattern Recognition (ICAITPR), ISBN 979-8-3503-6800-0, 2024.
Digital Object Identifier: 10.1109/ICAITPR63242.2024.10960115 [CrossRef]
[43] Evolutionary NetArchitecture Search for Deep Neural Networks Pruning, Chen, Shuxin, Lin, Lin, Zhang, Zixun, Gen, Mitsuo, Proceedings of the 2019 2nd International Conference on Algorithms, Computing and Artificial Intelligence, ISBN 9781450372619, 2019.
Digital Object Identifier: 10.1145/3377713.3377739 [CrossRef]
[44] LTS-NET: Lung Tissue Segmentation from CT Images using Fully Convolutional Neural Network, Zhou, Lingyu, Xu, Xiuyuan, Zhou, Kai, Guo, Jixiang, 2021 11th International Conference on Information Science and Technology (ICIST), ISBN 978-1-6654-1266-7, 2021.
Digital Object Identifier: 10.1109/ICIST52614.2021.9440594 [CrossRef]
[45] Brain Tumor Detection Using Image Segmentation, Raj, Krishna, Sharma, Ayushi, Sharma, Ravi, 2024 1st International Conference on Advances in Computing, Communication and Networking (ICAC2N), ISBN 979-8-3503-5681-6, 2024.
Digital Object Identifier: 10.1109/ICAC2N63387.2024.10894913 [CrossRef]
[46] Implant Segmentation in Radiographic Imagery Using Wavelet Decomposition and Multiresolution MTANN, Perwiratama, Rangga, Pranowo, Pranowo, Setyohadi, Djoko Budiyanto, 2021 9th International Conference on Information and Communication Technology (ICoICT), ISBN 978-1-6654-0447-1, 2021.
Digital Object Identifier: 10.1109/ICoICT52021.2021.9527440 [CrossRef]
[47] A Clustering Based Approach For Meningioma Tumors Extraction From Brain MRI Images, Sahoo, Akshya Kumar, Parida, Priyadarsan, 2020 IEEE International Symposium on Sustainable Energy, Signal Processing and Cyber Security (iSSSC), ISBN 978-1-7281-8880-5, 2020.
Digital Object Identifier: 10.1109/iSSSC50941.2020.9358849 [CrossRef]
[48] A survey of bone abnormalities detection using machine learning algorithms, Barhoom, Alaa M. A., Jubair, Mohammed Rasheed, Abu-Naser, Samy S., PROCEEDINGS OF THE 1ST INTERNATIONAL CONFERENCE ON FRONTIER OF DIGITAL TECHNOLOGY TOWARDS A SUSTAINABLE SOCIETY, ISBN , Issue , 2023.
Digital Object Identifier: 10.1063/5.0133139 [CrossRef]
[49] Deep neural networks for anatomical brain segmentation, de Brebisson, Alexandre, Montana, Giovanni, 2015 IEEE Conference on Computer Vision and Pattern Recognition Workshops (CVPRW), ISBN 978-1-4673-6759-2, 2015.
Digital Object Identifier: 10.1109/CVPRW.2015.7301312 [CrossRef]
[50] Machine Intelligence in Biomedical Data Modeling, Processing, and Analysis, Mujkic, Amar, Baralic, Ena, Ombasic, Aida, Becirovic, Lemana Spahic, Pokvic, Lejla Gurbeta, Badnjevic, Almir, 2022 11th Mediterranean Conference on Embedded Computing (MECO), ISBN 978-1-6654-6828-2, 2022.
Digital Object Identifier: 10.1109/MECO55406.2022.9797164 [CrossRef]
Disclaimer: All information displayed above was retrieved by using remote connections to respective databases. For the best user experience, we update all data by using background processes, and use caches in order to reduce the load on the servers we retrieve the information from. As we have no control on the availability of the database servers and sometimes the Internet connectivity may be affected, we do not guarantee the information is correct or complete. For the most accurate data, please always consult the database sites directly. Some external links require authentication or an institutional subscription.
Web of Science® is a registered trademark of Clarivate Analytics, Scopus® is a registered trademark of Elsevier B.V., other product names, company names, brand names, trademarks and logos are the property of their respective owners.
Faculty of Electrical Engineering and Computer Science
Stefan cel Mare University of Suceava, Romania
All rights reserved: Advances in Electrical and Computer Engineering is a registered trademark of the Stefan cel Mare University of Suceava. No part of this publication may be reproduced, stored in a retrieval system, photocopied, recorded or archived, without the written permission from the Editor. When authors submit their papers for publication, they agree that the copyright for their article be transferred to the Faculty of Electrical Engineering and Computer Science, Stefan cel Mare University of Suceava, Romania, if and only if the articles are accepted for publication. The copyright covers the exclusive rights to reproduce and distribute the article, including reprints and translations.
Permission for other use: The copyright owner's consent does not extend to copying for general distribution, for promotion, for creating new works, or for resale. Specific written permission must be obtained from the Editor for such copying. Direct linking to files hosted on this website is strictly prohibited.
Disclaimer: Whilst every effort is made by the publishers and editorial board to see that no inaccurate or misleading data, opinions or statements appear in this journal, they wish to make it clear that all information and opinions formulated in the articles, as well as linguistic accuracy, are the sole responsibility of the author.